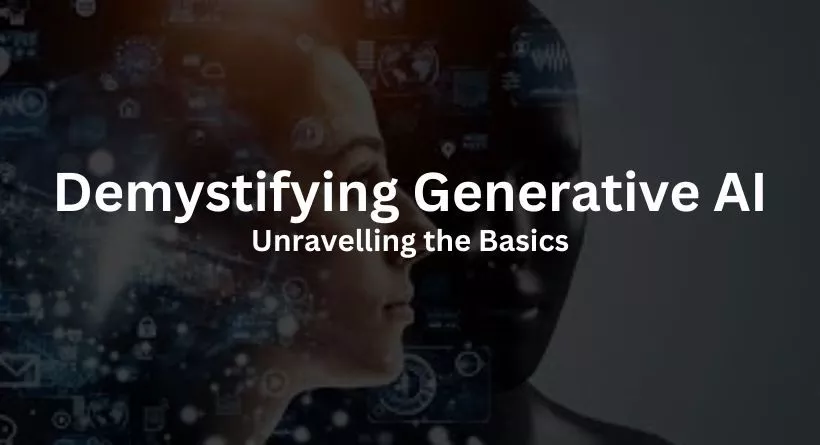
Artificial Intelligence (AI) can often seem like a complex maze, brimming with technical jargon and bewildering concepts. One term that has garnered considerable attention in recent years is “Generative AI.” In this article, we’ll demystify Generative AI, delve into its history, and elucidate the fundamental principles that underpin its development. All of this will be presented in a manner that anyone can grasp, irrespective of their technical background.
What is Generative AI?

At its core, Generative AI is a subset of AI techniques that can produce new content, encompassing a wide range of media such as images, text, music, and 3D models. Think of it as an intelligent, creative assistant capable of crafting unique and captivating content with minimal input from a human user. This feat is achieved through advanced machine learning models, which can discern patterns and generate fresh content based on their acquired knowledge.
You may also like reading: How to Navigate the Generative AI Hype Cycle
A Brief History of Generative AI
The roots of Generative AI can be traced back to the early days of AI research in the 1950s and 1960s. However, it wasn’t until the past decade that Generative AI made significant strides, thanks to advances in computational power and machine learning algorithms. A pivotal moment in the history of Generative AI was the creation of the Generative Adversarial Network (GAN) by Ian Goodfellow and his team in 2014. GANs consist of two neural networks, a generator, and a discriminator, working in a competitive synergy. The generator produces content, while the discriminator evaluates whether it’s genuine or generated. This process continues until the generator can create content indistinguishable from real examples, and GANs have been widely used to generate realistic images, videos, and other content.
Core Concepts of Generative AI
- Machine Learning: Machine learning involves training a computer model to identify patterns in data and make predictions based on those patterns. In Generative AI, machine learning models learn the underlying structure of a dataset, enabling them to create new content based on that structure.
- Neural Networks: Neural networks, inspired by the human brain, consist of interconnected nodes or “neurons” that collaborate to process input and generate output. Generative AI models often employ deep neural networks with multiple layers of neurons to grasp intricate data patterns.
- GANs (Generative Adversarial Networks): GANs involve two neural networks—a generator and a discriminator—competing to create realistic content.
- Reinforcement Learning: This type of machine learning involves an AI agent learning to make decisions by interacting with an environment and receiving feedback in the form of rewards or penalties. In Generative AI, reinforcement learning assists AI agents in mastering strategies for content creation.
Why Generative AI Matters
Generative AI holds the potential to revolutionize a multitude of industries, spanning marketing, entertainment, healthcare, and manufacturing. Here are a few ways Generative AI can be harnessed:
- Enhance Creativity: Generative AI models aid human creators in generating new ideas, designs, and solutions, accelerating the creative process and fostering innovation.
- Personalization: AI-generated content can be tailored to individual users, enhancing personalized experiences in areas such as advertising, content recommendations, and customer support, thereby increasing customer satisfaction and loyalty.
- Cost Savings: Generative AI can automate tasks that previously required human intervention, like content creation and data analysis, resulting in substantial cost savings and resource optimization.
- Improving Decision Making: By generating new insights and perspectives, Generative AI can facilitate more informed decision-making, leading to more efficient and effective business strategies.
- Addressing Resource Constraints: Generative AI enables businesses to overcome resource limitations by generating innovative solutions, designs, or products that might not have been achievable through conventional means.
Examples of Generative AI in Action
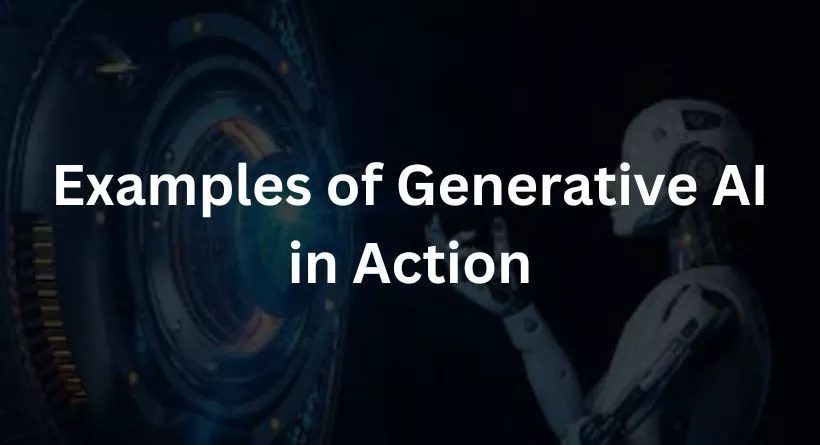
To illustrate the potential of Generative AI, here are some real-world examples:
1. Art and Design
Artists and designers are utilizing Generative AI tools to create one-of-a-kind artwork, fashion designs, and architectural concepts. These tools ignite creativity, streamline design processes, and push the boundaries of what’s achievable.
2. Drug Discovery
Pharmaceutical companies are leveraging Generative AI to expedite drug discovery by generating new drug candidates and predicting their efficacy. This can significantly reduce the time and cost of bringing new treatments to market.
3. Content Generation
Media companies and marketers are employing Generative AI to craft personalized content, such as news articles, video game environments, and marketing materials. This leads to enhanced user engagement and improved conversion rates.
4. Virtual Assistants
Generative AI is playing a pivotal role in developing advanced virtual assistants capable of understanding and responding to natural language queries, generating human-like responses, and engaging in conversations with users.
Conclusion
In conclusion, Generative AI is a groundbreaking field that promises to reshape various industries by enhancing creativity, personalization, and cost-effectiveness. Its core concepts, including machine learning, neural networks, GANs, and reinforcement learning, are pivotal to its functioning. As this technology continues to evolve, it’s important to stay informed about its applications and potential.
FAQs
What are the key applications of Generative AI?
Generative AI can be applied in diverse fields, including art and design, drug discovery, content generation, and the development of virtual assistants.
How does Generative AI benefit businesses?
Generative AI can help businesses by enhancing creativity, personalizing content, saving costs, improving decision-making, and addressing resource constraints.
What is the history of Generative AI?
Generative AI traces its roots back to the 1950s and 1960s but made significant advancements in the past decade with the development of GANs.
What is the role of neural networks in Generative AI?
Neural networks, particularly deep neural networks, are instrumental in Generative AI for understanding complex data patterns.
Can Generative AI be used in healthcare?
Yes, Generative AI has applications in healthcare, such as drug discovery and medical image analysis, which can lead to improved patient care.