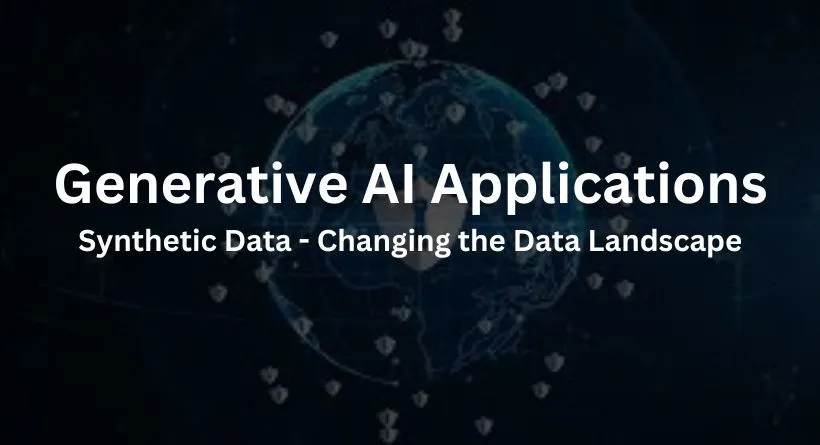
Welcome to the brave new world of data, a world that is not just evolving but also actively being reshaped by remarkable technologies.
It is a realm where our traditional understanding of data is continuously being challenged and transformed, paving the way for revolutionary methodologies and innovative tools.
The Data Evolution
Among these cutting-edge technologies, two stand out for their potential to dramatically redefine our data-driven future: Generative AI and Synthetic Data.
You may also like reading: Generative AI Applications: Procurement Revolution
What is Generative AI?
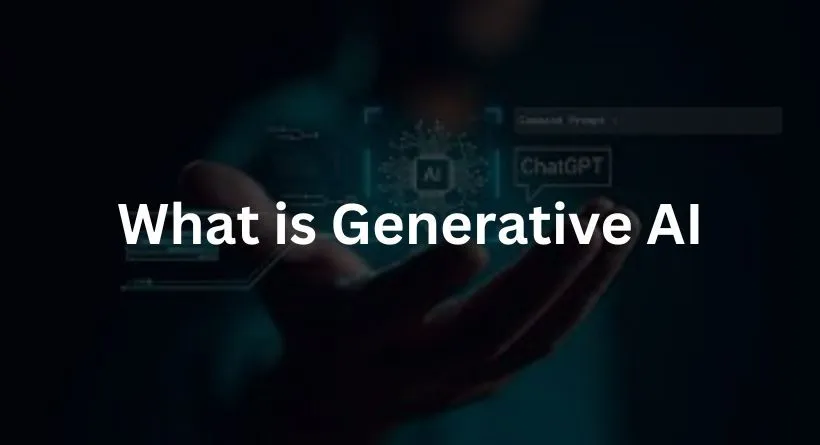
In this blog post, we will delve deeper into these fascinating concepts. We will explore what Generative AI and Synthetic Data are, how they interact, and most importantly, how they are changing the data landscape.
Generative AI Unveiled
So, strap in and get ready for a tour into the future of data.
Understanding Generative AI and Synthetic Data
The Power of Generative AI
Generative AI refers to a subset of artificial intelligence, particularly machine learning, that uses algorithms like Generative Adversarial Networks (GANs) to create new content. It’s ‘generative’ because it can generate something new and unique from random noise or existing data inputs, whether that be an image, a piece of text, data, or even music.
Generative Adversarial Networks (GANs)
GAN’s are powerful algorithms comprise two neural networks — the generator, which produces new data instances, and the discriminator, which evaluates them for authenticity. Over time, the generator learns to create more realistic outputs.
Advancements in Generative AI
Today, the capabilities of Generative AI have evolved significantly, with models like OpenAI’s GPT-4 showcasing a staggering potential to create human-like text. The technology is being refined and optimized continuously, making the outputs increasingly indistinguishable from real-world data.
What is Synthetic Data?
Synthetic data refers to artificially created information that mimics the characteristics of real-world data but does not directly correspond to real-world events. It is generated via algorithms or simulations, effectively bypassing the need for traditional data collection methods.
The Demand for Quality Data
In our increasingly data-driven world, the demand for high-quality, diverse, and privacy-compliant data is soaring.
Challenges with Real Data
Across industries, companies are grappling with data-related challenges that prevent them from unlocking the full potential of artificial intelligence (AI) solutions.
Data Regulations
These hurdles can be traced to various factors, including regulatory constraints, sensitivity of data, financial implications, and data scarcity.
Privacy and Transparency
Data regulations have placed strict rules on data usage, demanding transparency in data processing. These regulations are in place to protect the privacy of individuals, but they can significantly limit the types and quantities of data available for developing AI systems.
Sensitive Data
Moreover, many AI applications involve customer data, which is inherently sensitive. The use of production data poses significant privacy risks and requires careful anonymization, which can be a complex and costly process.
Financial Implications
Financial implications add another layer of complexity. Non-compliance with regulations can lead to severe penalties.
Data Availability
Furthermore, AI models typically require vast amounts of high-quality, historical data for training. However, such data is often hard to come by, posing a challenge in developing robust AI models.
The Role of Synthetic Data
This is where synthetic data comes in.
Synthetic Data Benefits
Synthetic data can be used to generate rich, diverse datasets that resemble real-world data but do not contain any personal information, thus mitigating any compliance risks. Additionally, synthetic data can be created on-demand, solving the problem of data scarcity and allowing for more robust AI model training.
Synthetic Data in Action
By leveraging synthetic data, companies can navigate the data-related challenges and unlock the full potential of AI.
What is Synthetic Data?
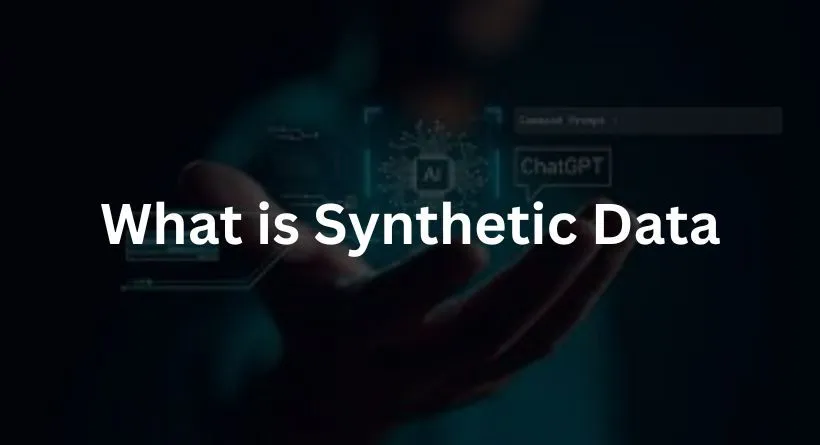
The Making of Synthetic Data
Synthetic data refers to data that’s artificially generated rather than collected from real-world events. It’s a product of advanced deep learning models, which can create a wide range of data types, from images and text to complex tabular data.
Characteristics of Synthetic Data
Synthetic data aims to mimic the characteristics and relationships inherent in real data, but without any direct linkage to actual events or individuals.
Anonymization and Compliance
A synthetic data generating solution can be a game-changer for complex AI models, which typically require massive volumes of data for training. These models can be “fed” with synthetically generated data, thereby accelerating their development process and enhancing their performance.
Privacy-Friendly Data
One of the key features of synthetic data is its inherent anonymization. Because it’s not derived from real individuals or events, it doesn’t contain any personally identifiable information (PII). This makes it a powerful tool for data-related tasks where privacy and confidentiality are paramount.
Compliance with Regulations
As such, it can help companies navigate stringent data protection regulations, such as GDPR, by providing a rich, diverse, and compliant data source for various purposes.
Transforming Data Landscape
In essence, synthetic data can be seen as a powerful catalyst for advanced AI model development, offering a privacy-friendly, versatile, and abundant alternative to traditional data. Its generation and use have the potential to redefine the data landscape across industries.
Synthetic Data Use Cases
Where Does Synthetic Data Shine?
Synthetic data finds significant utility across various industries due to its ability to replicate real-world data characteristics while maintaining privacy.
Testing and Development
Testing in Realistic Conditions
In Testing and Development, synthetic data can generate production-like data for testing purposes. This enables developers to validate applications under conditions that closely mimic real-world operations.
Quality Assurance in Machine Learning
Furthermore, synthetic data can be used to create testing datasets for machine learning models, accelerating the quality assurance process by providing diverse and scalable data without any privacy concerns.
Healthcare
Enhancing Medical Research
The health sector also reaps benefits from synthetic data. For instance, synthetic medical records or claims can be generated for research purposes, boosting AI capabilities without violating patient confidentiality.
Improving Diagnostic Accuracy
Similarly, synthetic CT/MRI scans can be created to train and refine machine learning models, ultimately improving diagnostic accuracy.
Financial Services
Secure Development
Financial Services can utilize synthetic data to anonymize sensitive client data, allowing for secure development and testing.
Fraud Detection Enhancement
Moreover, synthetic data can be used to enhance scarce fraud detection datasets, improving the performance of detection algorithms.
Insurance
Modeling Risk Scenarios
In Insurance, synthetic data can be used to generate artificial claims data. This can help in modeling various risk scenarios and aid in creating more accurate and fair policies, while keeping the actual claimant’s data private.
A World of Possibilities
These use cases are just the tip of the iceberg, demonstrating the transformative potential of synthetic data across industries.
Conclusion
In conclusion, the world of data is rapidly evolving, and Generative AI and Synthetic Data are at the forefront of this transformation. They offer innovative solutions to the challenges associated with real data, providing a path to unlock the full potential of artificial intelligence. As we move forward into this data-driven future, embracing these technologies will be crucial for staying competitive and compliant.
FAQs
1: What is the main advantage of using synthetic data in AI development?
Using synthetic data in AI development allows companies to access diverse and privacy-compliant data, overcoming the limitations and challenges associated with real data.
2: How does synthetic data ensure privacy and compliance with regulations?
Synthetic data is generated without any direct link to real individuals or events, making it inherently anonymized and compliant with data protection regulations.
3: Are there any limitations to the use of synthetic data?
While synthetic data offers many advantages, it may not fully capture the complexity of real-world data, making it suitable for certain use cases but not all.
4: What industries can benefit the most from synthetic data?
Industries such as healthcare, finance, insurance, and software development can benefit significantly from the use of synthetic data for testing, research, and development.
5: How can companies start incorporating synthetic data into their AI projects?
Companies can start by exploring synthetic data generation platforms and consulting with experts in the field to understand how it can best serve their specific AI project needs.