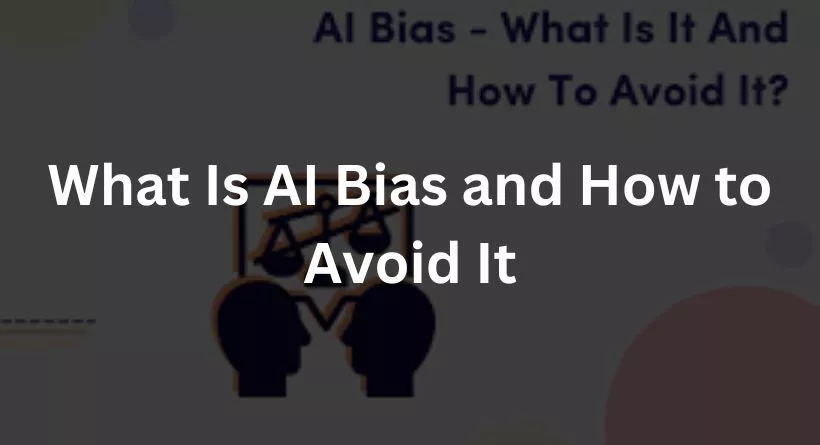
In an age where artificial intelligence is becoming increasingly integrated into our daily lives, the topic of AI bias has gained significant importance. Algorithms are not neutral when it comes to weighing people, events, or things differently for various purposes. Therefore, we must understand these biases so that we can develop solutions to create unprejudiced AI systems. This article will delve into what AI bias is, the different types of AI bias, provide examples, and discuss how to reduce the risk of AI bias.
What is AI Bias?
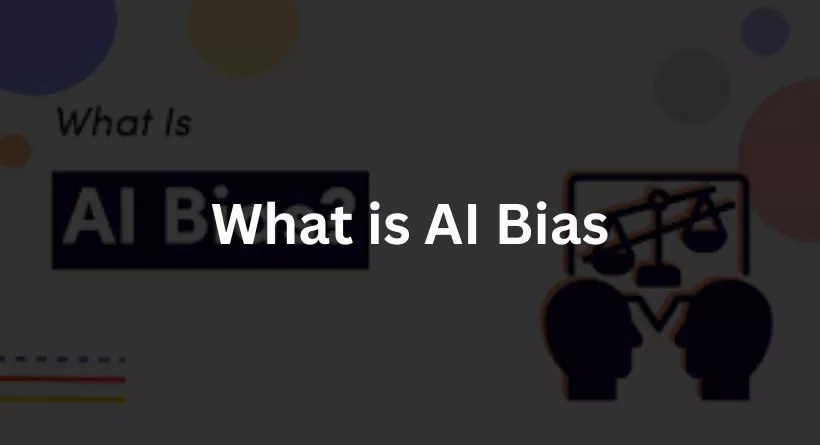
AI bias, also known as machine learning bias, algorithm bias, or artificial intelligence bias, refers to the tendency of algorithms to reflect human biases. It is a phenomenon that arises when an algorithm delivers systematically biased results as a consequence of erroneous assumptions made during the machine learning process. In today’s climate of increasing representation and diversity, this issue becomes even more problematic, as algorithms could be reinforcing biases.
You may also like reading: How to Get Benefits of AI in 2023: Top Applications of AI in Business
For instance, consider a facial recognition algorithm that has been trained predominantly on data of white individuals. This algorithm may perform better at recognizing white faces than faces of individuals from minority groups, such as black people. This unintentional bias can negatively affect minority groups, perpetuating discrimination and hindering equal opportunities. The challenge with these biases is that they are often hard to detect until they have been programmed into the software.
3 AI Bias Examples
To illustrate the real-world impact of AI bias, let’s explore three examples:
1. Racism in the American Healthcare System
In 2019, researchers discovered that an algorithm used in US hospitals to predict which patients would require additional medical care favored white patients over black patients by a significant margin. This algorithm’s bias was rooted in the patients’ past healthcare expenditures, which was significantly related to their race. Black individuals with similar health conditions had spent less on healthcare than their white counterparts. Researchers and a health services company collaborated to reduce the bias, highlighting the importance of addressing these issues.
2. Depicting CEOs as Purely Male
Studies have shown that image search results for the term “CEO” often display predominantly male figures, despite an increasing number of female CEOs in the United States. This bias in image search results perpetuates stereotypes and can influence how society perceives leadership roles.
3. Amazon’s Hiring Algorithm
Amazon’s experimental recruiting tool used AI to rate job applicants from one to five stars. However, it was found to be biased towards women, penalizing female applicants and demoting applications from graduates of all-female institutions. The necessary changes were made when the bias was detected, but it raised concerns about AI biases affecting hiring decisions.
How AI Bias Reflects Society’s Biases
AI is susceptible to human bias just like everyone else. If we put a lot of effort into ensuring sure AI systems are fair, it can help humans make judgements that are more objective. AI bias is frequently caused by the underlying data rather than the AI algorithms themselves. Several elements have been highlighted by McKinsey research as contributing to AI bias:
- Models trained on data from human choices or data reflecting social disparities may perpetuate biases.
- Data collection methods and selection processes can introduce bias.
- User-generated data may create a feedback loop that reinforces bias.
- Machine learning systems can detect statistically inappropriate or unlawful connections, such as age-based discrimination.
One example is the Apple credit card, which offered significantly different credit limits to male and female applicants, illustrating the potential consequences of AI bias.
What Can We Do About the Biases in AI?
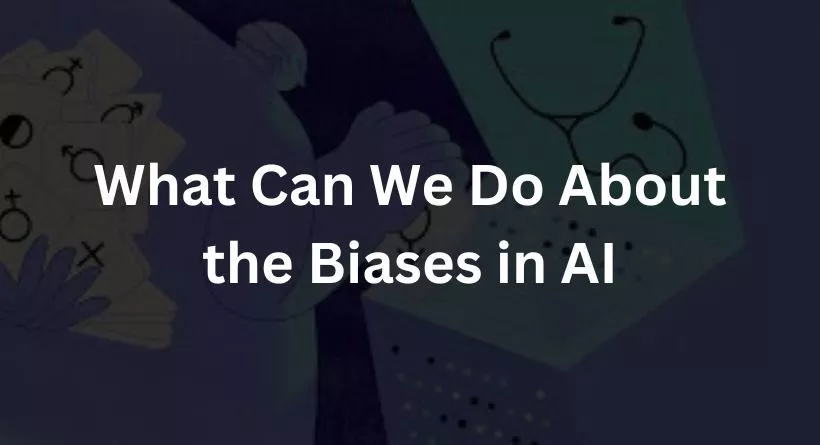
Addressing AI bias is essential to ensure fairness and equity in AI systems. Here are some proposed solutions:
1. Testing Algorithms in Real-Life Settings
Testing AI algorithms in scenarios that resemble real-world usage is crucial. This helps identify and rectify biases that may not be evident when applied to a specific group of data but could affect different groups.
2. Accounting for Counterfactual Fairness
The definition of fairness varies, and it can change due to external factors. AI systems should consider these variations and adapt to ensure fairness in diverse contexts.
3. Implementing Human-in-the-Loop Systems
Human-in-the-Loop technology involves human intervention when AI systems cannot make unbiased decisions. This approach leads to continuous feedback, improving accuracy and fairness over time.
4. Changing the Way We Educate About Science and Technology
Reforming education in science and technology is essential to addressing AI bias. A multidisciplinary approach involving ethicists, social scientists, and humanities scholars is needed to tackle complex issues related to AI bias.
Will AI ever be unbiased?
The quick response? Indeed and no. Although it’s doubtful, it’s not impossible that there will ever be a completely objective AI. This is because it is improbable that there can ever be a completely objective human consciousness. The quality of the data that artificial intelligence systems are fed is what determines how good the system will be. Assume you are able to remove any conscious and unconscious biases related to gender, ethnicity, and other ideologies from your training dataset. In such scenario, you will be able to develop an artificial intelligence system that renders unbiased decisions based on facts.
On the other hand, we are aware that this is improbable in real life. The data that AI is given and learns from shapes its decisions. The data that AI consumes is generated by humans. Humans are biased in various ways, and the number of biases grows steadily as new biases are consistently discovered. It is therefore possible that neither an AI system nor a completely objective human consciousness will ever be attained. Humans are the ones who create the skewed data, and humans and algorithms created by humans are the ones that validate the data to identify and fix biases.
FAQs
1. What is AI bias?
AI bias, also known as algorithm bias, refers to the tendency of algorithms to reflect human biases, resulting in systematically biased results.
2. How can AI bias be reduced?
AI bias can be reduced through testing algorithms in real-life settings, accounting for counterfactual fairness, implementing human-in-the-loop systems, and changing the way we educate about science and technology.
3. Why is AI bias a critical issue?
AI bias can perpetuate discrimination, hinder equal opportunities, and impact various aspects of society, from healthcare to hiring decisions.
4. What is counterfactual fairness in AI?
Counterfactual fairness ensures that an AI model’s decisions remain the same even when sensitive characteristics, such as race or gender, are altered in a hypothetical scenario.
5. How can we ensure fairness in AI systems?
Ensuring fairness in AI systems requires addressing bias in data, testing algorithms rigorously, and involving diverse perspectives in the development and deployment of AI technologies.